We are at the doorstep of an energy crisis trilemma- security, affordability, and sustainability of power generation and distribution sources. Furthermore, exogeneous factors like weather fluctuation can also put the national energy system under pressure. Limited non-renewable stock, insufficient renewable storage, local market disturbances and relatively low investment in the hydrocarbon sector causes additional imbalance. Such fiscal stresses limit the timely accomplishment of investment programs for smooth energy transitions. However, as simple as it may sound, energy transition is neither easy and cheap, nor stable at the moment.
One of the rapid responses towards energy transition is the clean baseload of power generation. Take Italy, for example. It is one of the leading countries within the EU in terms of harnessing wind and solar power. However, energy prices are surging for the end-user and providers are trapped in finding energy transition solution must adhere to the net-zero policies.
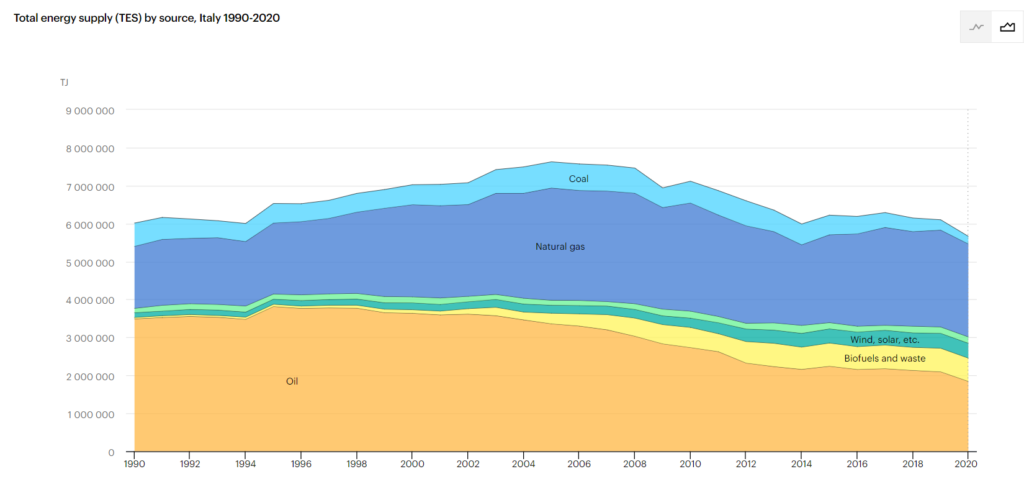
In fact, the cost of power generation depends not only the feed-in tariffs but also on plant capacity. This is where technologists help by maximizing asset availabilities, forecasting potential faults/failures, proposing reliable response time to asset interventions etc. In short, this is where the difference between maintenance and reliability can make a huge difference.
Firstly, maintenance and reliability are NOT the same thing. Just because something is well-maintained does not mean it is reliable. If something has been reliable up until now does not mean you can slack on the maintenance part of it. Interestingly, the root cause that makes people to often interchange these terms on the basis of “life-extension” guarantee. As ironic as it may sound right now, life-extension itself is a misnomer. We often talk about the bathtub curve of asset reliability. The more resource we spend at maintenance the more we expect the curve to elongate. But is that how it really works?
Companies want to develop risk functions by evolving governance, policies, and tech to increase uptime and regulate costs. Typically, risk is defined as the product of probability and consequences of an event occurring during the asset lifetime. Depending upon the severity of consequences risk factor can also increase exponentially. It is evident that in such models, continuous availability of updated, integrable and reliable data is of essence.
A risk function for the energy sector can identify asset improvement opportunities using overall asset health status. This can enable criticality analysis and definitely become a decision support tool. It can reduce negative consequences of unplanned downtime and provide better control in resource allocation for the service provider.
This is where users should look at asset indexing strategies, similar to transformer health indexing models. Using diagnostic testing data and loss-inducing parameters, a combined transformer health-risk indexing model can be certainly developed. Dissolved gas analysis and moisture readings can give almost 50% insights to incipient faults in windings, bushings, and tap-changers. This means data indicating the transformer (or any asset by extension) health can be consolidated into a single sheet. It will allow calculation subsequent maintenance and downtime costs. Service providers can re-adopt to best practices for their plant/asset. It will create transparency, highlight and rank risky assets in a fleet for better management of aged population. Ultimately, it can better justify resource allocation and refurbishing/replacement costs.
Therefore, it is time to adopt to smart analytics and indexing functions using artificial intelligence to solve energy transition challenges.